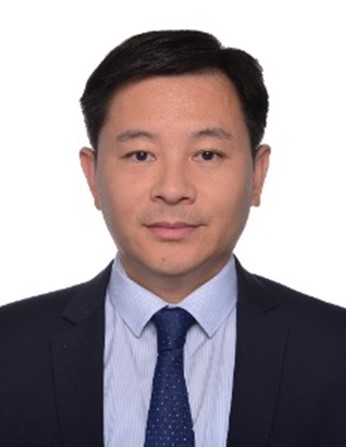
Speaker: Shengbo Eben Li, Tsinghua University, China
Title: Safe Control Policy and Feasible Set in Constrained Reinforcement Learning.
Abstract: Safety is one of the major focuses of real-world dynamical systems. Safety of the dynamical system means that the system must lie in the feasible set. The feasible sets are usually unknown subsets of the set of safe states, which makes the controller design difficult. In this talk, I will introduce reinforcement learning algorithms that can jointly learn the feasible set and the optimal safe control policy. The feasible sets are modeled by safety index or reachability, then we use adversarial learning to find the feasible set and safe control policies.
Biography: Shengbo Eben Li received his M.S. and Ph.D. degrees from Tsinghua University in 2006 and 2009. Before joining Tsinghua University, he has worked at Stanford University, University of Michigan, and UC Berkeley. His active research interests include intelligent vehicles and driver assistance, deep reinforcement learning, optimal control and estimation, etc. He is the author of over 190 peer-reviewed journal/conference papers, and co-inventor of over 40 patents. Dr. Li has received over 20 prestigious awards, including Youth Sci. & Tech Award of Ministry of Education (annually 10 receivers in China), Natural Science Award of Chinese Association of Automation (First level), National Award for Progress in Sci & Tech of China, and best (student) paper awards of IET ITS, IEEE ITS, IEEE ICUS, CVCI, etc. He also serves as Board of Governor of IEEE ITS Society, Senior AE of IEEE OJ ITS, and AEs of IEEE ITSM, IEEE TITS, IEEE TIV, IEEE TNNLS, etc.
Speaker: Yujie Tang, Peking University, China
Title: Benign Nonconvex Landscapes in Optimal and Robust Control
Abstract: Direct policy search has achieved great empirical success in reinforcement learning. Many recent studies have revisited its theoretical foundation for continuous control, which reveals elegant nonconvex geometry in various benchmark problems, especially in fully observable state-feedback cases. In this talk, we start from two fundamental control problems with partial observability, namely linear quadratic Gaussian control and H-infinity robust control, and investigate the landscape properties of their policy optimization formulations. Despite the lack of convexity (and possibly smoothness) of the cost functions, our main results show that for a class of non-degenerate policies, all Clarke stationary points are globally optimal and there is no spurious local minimum for both LQG and H-infinity control. Our proof techniques rely on a new and unified framework of Extended Convex Lifting (ECL), which reconciles the gap between nonconvex policy optimization and convex reformulations. We will then provide an introduction to the ECL framework, with a focus on its underlying geometric intuitions and its applicability to a wide range of classical control problems. We hope that our proposed ECL framework will further promote understanding of the hidden convexity of nonconvex policy optimization for classical control problems.
Biography: Yujie Tang received the Bachelor's degree in Electronic Engineering from Tsinghua University, China in 2013, and the Ph.D. degree in Electrical Engineering from the California Institute of Technology, USA in 2019. From 2019 to 2022, he worked as a postdoctoral fellow in the School of Engineering and Applied Sciences at Harvard University. Since 2022, he has served as an assistant professor in the Department of Industrial Engineering and Management at Peking University. His research interests include distributed optimization and zeroth-order optimization, reinforcement learning and data-driven control methods, and optimization methods for power and energy systems.
Speaker: Gang Wang, Beijing Institute of Technology, China
Title: Data-driven Control and Observers for Networked Systems
Abstract: In recent years, the evolution of Big Data and AI technology has led to the gradual adoption of end-to-end direct data-driven control, replacing traditional identification-based control methods (a.k.a. indirect data-driven control). This shift has garnered significant attention from both the control systems and machine learning communities. This talk begins with a brief overview of data-driven control rooted in behavioral system theory. It delves into the exploration of data-driven resilient control strategies for unknown networked systems vulnerable to denial-of-service (DoS) attacks, alongside design and analysis of data-driven unknown-input observers (UIO), as well as data-driven linear quadratic Gaussian (LQG) controllers. Further, it addresses generalization to distributed and multi-agent settings. Concluding, this talk highlights applications of data-driven predictive control techniques in enabling agile flight for quadrotors.
Biography: Dr. Gang Wang is currently a Professor in the School of Automation at Beijing Institute of Technology. He earned dual Ph.D. degrees from the University of Minnesota and Beijing Institute of Technology in 2018. Following this, from 2018 to 2020, he served as a postdoctoral researcher at the University of Minnesota. Dr. Wang's research interests include data-driven control and world model predictive control for quadrotors. He has authored 70 papers in top IEEE Transactions such as IEEE TIT/TAC/TSP, in addition to 60 conference papers at venues including NeurIPS, AISTATS, CDC, and ICRA. Dr. Wang has received several awards, including the IEEE SPS Outstanding Editorial Board Member Award in 2023, IEEE CSS Beijing Chapter Young Author Prize in 2022, FITEE Best Paper Award in 2021, IEEE PES-GM Best Paper Award in 2019, EUSIPCO Best Student Paper Award in 2017, and the CAA Outstanding Ph.D. Thesis Award in 2020. He presently serves on the editorial boards of Signal Processing and IEEE Transactions on Signal and Information Processing over Networks, as well as the IEEE Open Journal of Control Systems.
Speaker: Keyou You, Tsinghua University, China
Title:Minimum Sample Data for Direct Data-driven Analysis and Adaptive LQR Design of Unknown Linear Systems
Abstract: Modern control theory has been firmly rooted in the state-space model, and then adopts system identification (SysId) followed by model-based control design methods. In this talk, we are motivated by two questions that possibly promote rethinking of this foundation: (a) whether SysId is indispensable to control design, and (b) if not, can we address it in a direct data-driven fashion (bypassing the SysId step)? In particular, via a new concept of sufficient richness of input sectional data, we first establish the necessary and sufficient condition for the minimum sample data for property ID (system analysis) of unknown linear systems. Specifically, the input sectional data is sufficiently rich for property ID if and only if it spans a linear subspace that contains a property dependent minimum linear subspace, any basis of which can also be easily used to form the minimum excitation input. Interestingly, we show that many structural properties can be identified with the minimum input that is however unable to complete SysId. Then, we propose an optimal data-enabled LQR formulation in the sense of achieving minimum regret of the quadratic cost, and design a novel data-enabled policy optimization (DeePO) method using only a batch of online persistently exciting (PE) data. Finally, we numerically validate the theoretical results and demonstrate the computational and sample efficiency of our method.
Biography: Keyou You received the B.S. degree in Statistical Science from Sun Yat-sen University, Guangzhou, China, in 2007 and the Ph.D. degree in Electrical and Electronic Engineering from Nanyang Technological University (NTU), Singapore, in 2012. After briefly working as a Research Fellow at NTU, he joined Tsinghua University in Beijing, China where he is now a Full Professor in the Department of Automation. He held visiting positions at Politecnico di Torino, Hong Kong University of Science and Technology, University of Melbourne and etc.
Prof. You’s research interests focus on the intersections between control, optimization and learning as well as their applications in autonomous systems. He received the Guan Zhaozhi award at the 29th Chinese Control Conference in 2010 and the ACA (Asian Control Association) Temasek Young Educator Award in 2019. He received the National Science Funds for Excellent Young Scholars in 2017, and for Distinguished Young Scholars in 2023. Currently, he is an Associate Editor for Automatica, IEEE Transactions on Control of Network Systems, and IEEE Transactions on Cybernetics.